EDIT: This whole article should’ve been one sentence: “specialists aren’t generalists, big company MLEs are specialists, startups need generalists, act accordingly“
all of my advice is under-qualified rambling
ChatGPT says sharpshooting is the Olympic sport in which participants demonstrate exceptional accuracy in firing firearms or other projectile weapons. A sharpshooter is someone who takes part in such sports.
Sharpshooters are highly specialized, and their goal is to increase accuracy.
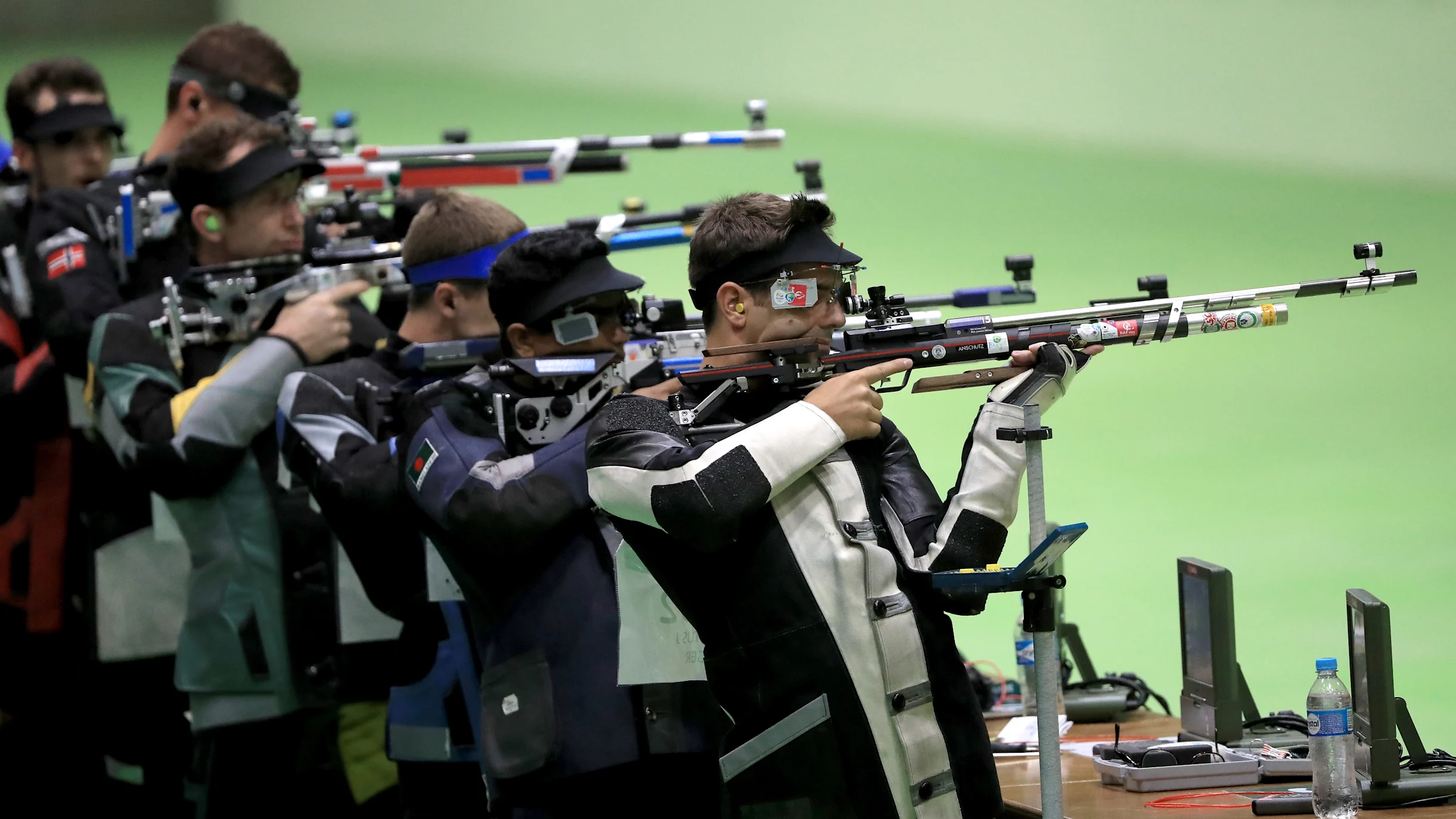
.png)
On the other hand, a sniper is a military or paramilitary marksman who engages targets from concealed positions or at distances that exceed the target's detection capabilities.
While in popular media people think that a sniper’s goal is to be accurate - most of the time the real goal of a sniper is to “make sure your team gets home safe” (or at least, in my totally unqualified mind and for the purpose of the analogy, that’s what i believe.
BigCo Data vs Startup Data
Academic researchers, MLEs at big companies, are like sharpshooters. 8 digit budgets a year across an enterprise to spend on compute to train massive models on petabytes of data. You’re measured by agreed upon criteria - in relatively controlled environments, by a judgement criteria. You never need to think about profits or costs - just solving the next highly specialized and technically difficult engineering problem. A 1% improvement on targeting accuracy is another $50M in revenue due to the 5% increase in conversions. You’re like a quant at jane street figuring out the right arbitrage to pour ad-money into to get revenue and profits out.
Your job is so valuable - bc your single digit percentage improvements are so valuable… that any time someone distracts you from the big problem, you SHOULD be saying “that’s not my job”.
Being a data scientist or MLE or whatever at a startup is a categorically different game. Your company is default dead. 10% increase in accuracy is a rounding error. Problems aren’t hard - there are just 10 of them so you need to find the 80/20 in 10% of the time and you might still not be doing enough.
Your success isn’t measured in accuracy or precision - it’s measured in whether or not your company goes bankrupt and everyone loses their job. At a startup, life is not a given - and you don’t have the luxury of optimizing. Even thinking about optimizing before something is already on fire is by definition over-optimization. You might be called on to close a deal. You might be called on to make some changes to the front end. “That’s not my job“ isn’t part of your vocabulary at all.
If you’re at BigCo
Don’t join a startup if you want to stay at the cutting edge of whatever your domain of optimization is. I don’t know the stats - but if I had to guess, military snipers don’t do very well at the olympics. It’s nice to tell ourselves that if you can do all the general tasks - that toughens you up to do the specialist tasks even better.
This is not true. If you want to be a published researcher - stay in the environment where you’re so highly leveraged, companies will buy software to keep you in the zone.
.png)
If you’re hiring for a data scientist at a startup
Don’t hire from big tech. They’re not going to want what you offer - and if you manage to convince them to, they’ll be sorely disappointed once they start. “What do you mean I can’t query a petabyte of data whenever I want? - wait we don’t even have a terabyte of data to train on?”
Unless…
I was going to write a whole inspirational thing about going into the arrangement with eyes wide open - aware of the tradeoffs on both sides… but I realized that this is already getting a little too preachy for my taste.
Just be honest with yourselves. A startup isn’t going to give you the power to do what you get to do at a company that spends 7 figures and up on compute for ML training. A startup is a place where every day is a fight to stay alive.
And as long as you’re honest with yourself everything will be fine
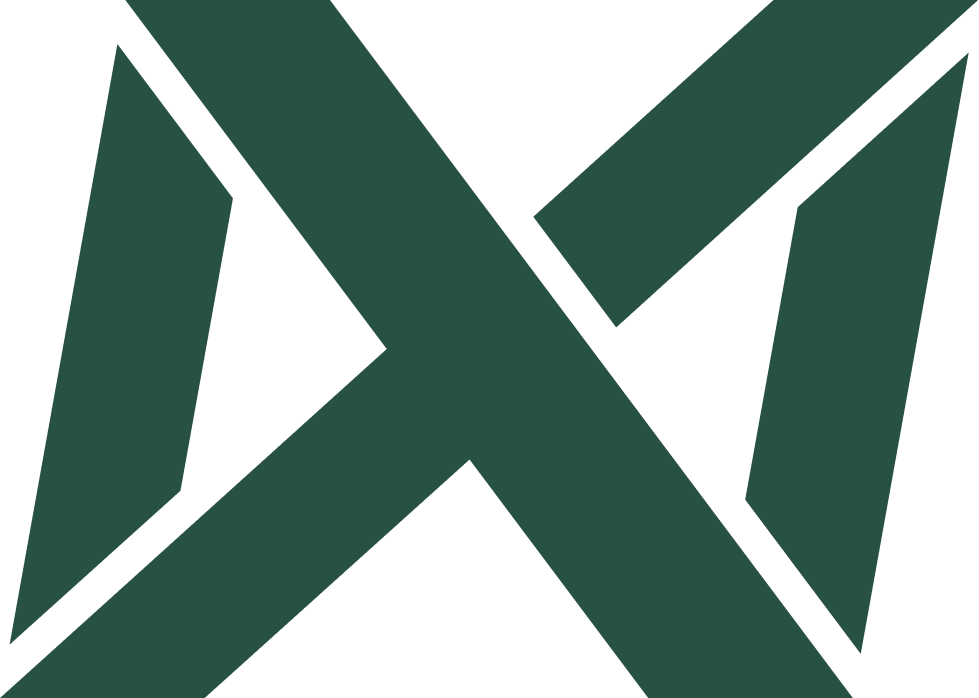
Stay Informed with TextQL's Newsletter
Sign up to receive updates on product news and future blog posts.
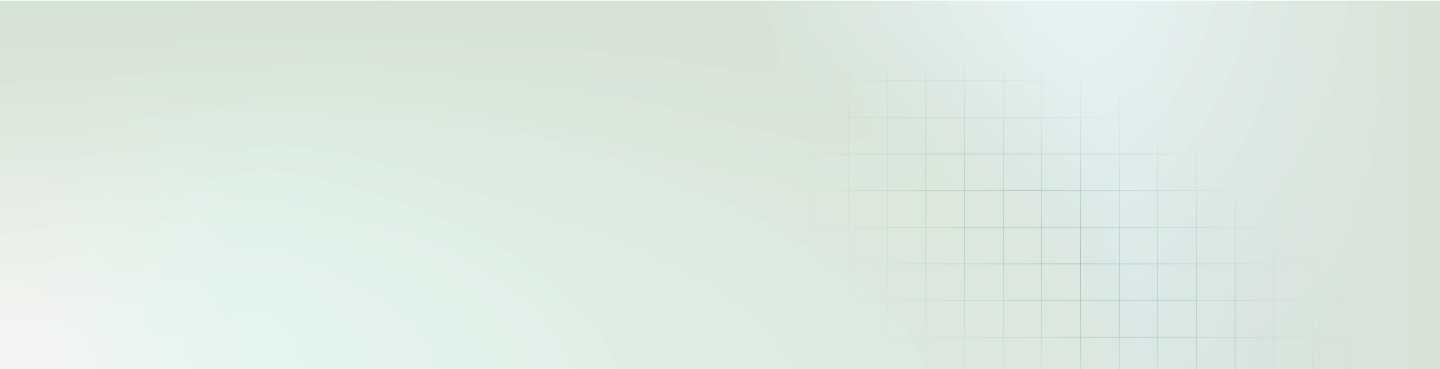